DTI_PANORAMA
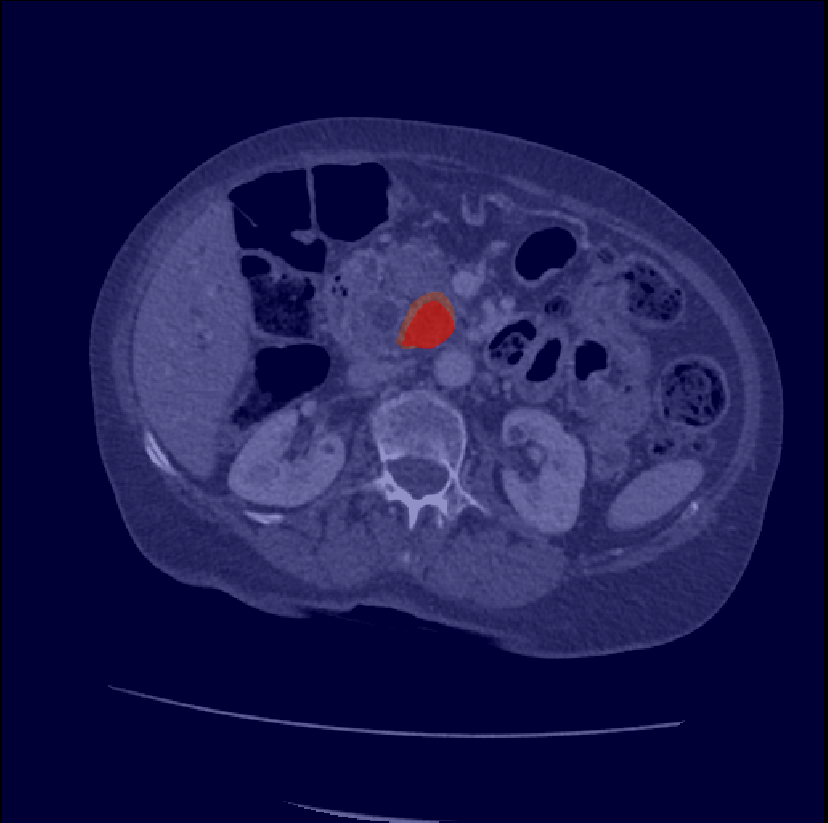
About
Interfaces
This algorithm implements all of the following input-output combinations:
Inputs | Outputs | |
---|---|---|
1 |
Challenge Performance
Date | Challenge | Phase | Rank |
---|---|---|---|
Oct. 3, 2024 | PANORAMA | Development Phase - Sanity Check | 1 |
Jan. 23, 2025 | PANORAMA | Development Phase - Tuning | 1 |
Feb. 25, 2025 | PANORAMA | Testing Phase | 1 |
Model Facts
Summary
Pancreatic ductal adenocarcinoma (PDAC) is one of the most common and aggressive types of pancreatic cancer. However, due to the lack of early and disease-specific symptoms, most patients with PDAC are diagnosed at an advanced disease stage. Consequently, early PDAC detection is crucial for improving patients’ quality of life and expanding treatment options. In this work, we develop a coarse-to-fine approach to detect PDAC on contrast-enhanced CT scans. First, we localize and crop the region of interest from the low-resolution images, and then segment the PDAC-related structures at a finer scale. Additionally, we develop two strategies to further boost the detection performance, including (1) a data splitting strategy for model ensembling, and (2) a customized post-processing function. We participated in the PANORAMA challenge1 and ranked 1st place for PDAC detection with an AUROC of 0.9263 and an AP of 0.7243. Our code and models are publicly available at https://github.com/han-liu/PDAC_detection.
Mechanism
Please refer to our technical report: AI-assisted Early Detection of Pancreatic Ductal Adenocarcinoma on Contrast-enhanced CT (https://arxiv.org/pdf/2503.10068)
Validation and Performance
We ranked 1st place in the testing phase of the PANORAMA challenge, with an AUROC of 0.9263 and an AP of 0.7243. More details can be found in our technical report.
Uses and Directions
This algorithm was developed for research purposes only.
Warnings
Common Error Messages
If you have any questions, feel free to open an issue at our GitHub page: https://github.com/han-liu/PDAC_Detection
Information on this algorithm has been provided by the Algorithm Editors, following the Model Facts labels guidelines from Sendak, M.P., Gao, M., Brajer, N. et al. Presenting machine learning model information to clinical end users with model facts labels. npj Digit. Med. 3, 41 (2020). 10.1038/s41746-020-0253-3