Breast and fibroglandular tissue segmentation
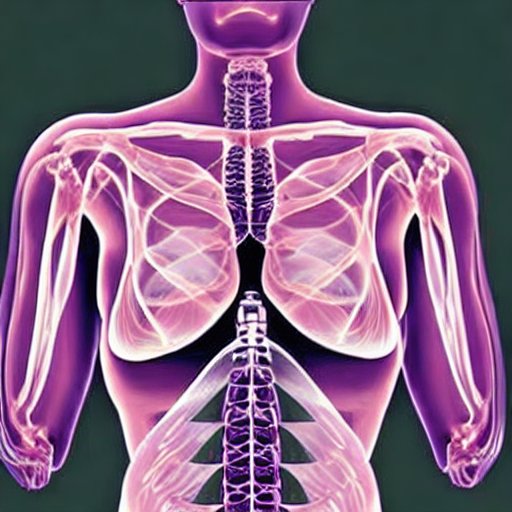
About
- T1 MRI (Any T1 weighted MRI)
- Breast and FGT Segmentation (A breast and fibroglandular tissue segmentation map)
Model Facts
Summary
This algorithm produces a volumetric segmentation mask for the fatty tissue and the fibroglandular tissue (FGT) in the breast given as input either a fat (FS) or without fat suppression (WOFS) T1 breast MR acquisition. The output of this algorithm allows to easily calculate breast density from a FS or WOFS T1 breast MR acquisition.
Mechanism
The algorithm is a fully automated deep learning segmentation network. The network architecture is based on the nnUNet framework.
For more details, please refer to our article: Exploiting the Dixon Method for a Robust Breast and Fibro-Glandular Tissue Segmentation in Breast MRI
Validation and Performance
We compared a network trained with only without fat-suppression (WOFS-only) T1 breast MR acquisitions and a network trained with both WOFS and FS (WOFS + FS) T1 breast MRI acquisitions. Below you can find the Dice Similarity Coefficient (DSC) on 9 held-out test cases from our internal dataset for both networks.
Network trained on | Test | Breast DSC [95% CI] | FGT DSC [95% CI] |
---|---|---|---|
WOFS-only | WOFS | 0.96 [0.94, 0.97] | 0.92 [0.89, 0.95] |
FS | 0.10 [0.08, 0.12] | 0.15 [0.11, 0.19] | |
WOFS + FS | WOFS | 0.96 [0.95, 0.97] | 0.91 [0.87, 0.94] |
FS | 0.95 [0.94, 0.96] | 0.86 [0.82, 0.91] |
Uses and Directions
This algorithm was developed for research purposes only.
Warnings
Common Error Messages
Information on this algorithm has been provided by the Algorithm Editors, following the Model Facts labels guidelines from Sendak, M.P., Gao, M., Brajer, N. et al. Presenting machine learning model information to clinical end users with model facts labels. npj Digit. Med. 3, 41 (2020). 10.1038/s41746-020-0253-3