Colon Budding in IHC
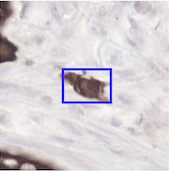
About
- Generic Medical Image
- Generic Overlay (An overlay of unknown type. Legacy, please use alternative interfaces.)
Model Facts
Summary
Tumor budding is a histopathological biomarker associated with metastases and adverse survival outcomes in colorectal carcinoma (CRC) patients. It is characterized by the presence of single tumor cells or small clusters of cells within the tumor or at the tumor-invasion front. In order to obtain a tumor budding score for a patient, the region with the highest tumor bud density must first be visually identified by a pathologist, after which buds will be counted in the chosen hotspot field. The automation of this process will expectedly increase efficiency and reproducibility. Here, we present a deep learning convolutional neural network model that automates the above procedure. For model training, we used a semi-supervised learning method, to maximize the detection performance despite the limited amount of labeled training data. The model was tested on an independent dataset in which human- and machine-selected hotspots were mapped in relation to each other and manual and machine detected tumor bud numbers in the manually selected fields were compared. We report the results of the proposed method in comparison with visual assessment by pathologists. We show that the automated tumor bud count achieves a prognostic value comparable with visual estimation, while based on an objective and reproducible quantification. We also explore novel metrics to quantify buds such as density and dispersion and report their prognostic value. We have made the model available for research use on the grand-challenge platform.
Citation: Bokhorst, John-Melle et al. "Semi-Supervised Learning to Automate Tumor Bud Detection in Cytokeratin-Stained Whole-Slide Images of Colorectal Cancer" Cancers (2023)
Note that the hotspots are manually selected in this example.
Contact information¶
Questions related to this algorithm can be addressed to John-Melle Bokhorst
Additional information¶
Please take the following limitations into account when submitting data to this algorithm: All input images should be in .tif format and contain magnification levels that correspond to 0.48μm pixel spacing (± 0.05). The algorithm will stop if any of the input images miss one of these levels.
Mechanism
When a Whole Slide Image (WSI) is uploaded, the algorithm automatically detects all tumor buds in the provided WSI using the developed Faster-RCNN network.
The result can be downloaded or viewed online.
Result will contain: - Tumor buds - Non tumor buds
Validation and Performance
Sensitivity | |
---|---|
Automatic detections | 0.91 |
Uses and Directions
This algorithm was developed for research purposes only. The terms of usage for Grand Challenge apply to the uploaded slides.
Warnings
Common Error Messages
Information on this algorithm has been provided by the Algorithm Editors, following the Model Facts labels guidelines from Sendak, M.P., Gao, M., Brajer, N. et al. Presenting machine learning model information to clinical end users with model facts labels. npj Digit. Med. 3, 41 (2020). 10.1038/s41746-020-0253-3