Colon Tissue segmentation
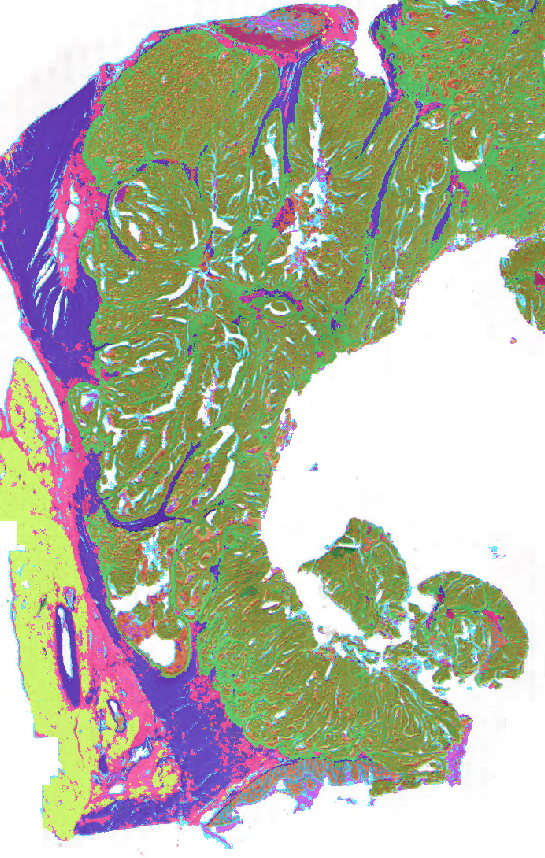
About
- Generic Medical Image
- Generic Overlay (An overlay of unknown type. Legacy, please use alternative interfaces.)
Model Facts
Summary
In colorectal cancer (CRC), artificial intelligence (AI) can alleviate the laborious task of characterization and reporting on resected biopsies, including polyps, the numbers of which are increasing as a result of CRC population screening programs ongoing in many countries all around the globe. Here, we present an approach to address two major challenges in the automated assessment of CRC histopathology whole-slide images. We present an AI-based method to segment multiple 14 tissue compartments in the H&E-stained whole-slide image, which provides a different, more perceptible picture of tissue morphology and composition. We test and compare a panel of state-of-the-art loss functions available for segmentation models, and provide indications about their use in histopathology image segmentation, based on the analysis of a) a multi-centric cohort of CRC cases from five medical centers in the Netherlands and Germany, and b) two publicly available datasets on segmentation in CRC. We used the best performing AI model as the basis for a computer-aided diagnosis system that classifies colon biopsies into four main categories that are relevant pathologically. We report the performance of this system on an independent cohort of more than 1,000 patients. The results show that with a good segmentation network as a base, a tool can be developed which can support pathologists in the risk stratification of colorectal cancer patients, among other possible uses.
Contact information¶
Questions related to this algorithm can be addressed to John-Melle Bokhorst
Mechanism
The result can be downloaded or viewed online.
Result will contain: 1. - Normal epithelium 2. - Low-grade dysplastic (LGD) epithelium 3. - High-grade dysplastic (HGD)/cancerous epithelium 4. - Stroma lamina propria 5. - Submucosal stroma 6. - Desmoplastic stroma 7. - Muscle 8. - Nerve 9. - Adipose 10. - Mucus 11. - Necrosis & debris 12. - Background
Additional information¶
Please take the following limitations into account when submitting data to this algorithm: All input images should be in .tif format and contain magnification levels that correspond to 1.0μm pixel spacing (± 0.05). The algorithm will stop if any of the input images miss one of these levels.
Validation and Performance
Class | DICE |
---|---|
Dysplasia High grade / Tumor | 0.83 |
Dysplasia low grade | 0.77 |
Normal Glands | 0.88 |
Desmoplastic Stroma | 0.58 |
Lamina propria | 0.82 |
Submucosal Stroma | 0.54 |
Necrosis and Debris | 0.45 |
Lymphocytes | 0.84 |
Erythrocytes | 0.68 |
Muscle | 0.82 |
Adipose tissue | 0.86 |
Mucus | 0.62 |
Nerve | 0.83 |
Background | 0.51 |
--------- | -------- |
Average | 0.72 |
Uses and Directions
This algorithm was developed for research purposes only. The terms of usage for Grand Challenge apply to the uploaded slides.
Warnings
Common Error Messages
Information on this algorithm has been provided by the Algorithm Editors, following the Model Facts labels guidelines from Sendak, M.P., Gao, M., Brajer, N. et al. Presenting machine learning model information to clinical end users with model facts labels. npj Digit. Med. 3, 41 (2020). 10.1038/s41746-020-0253-3