Lung cancer risk estimation on thorax CT scans - DSB2017 grt123
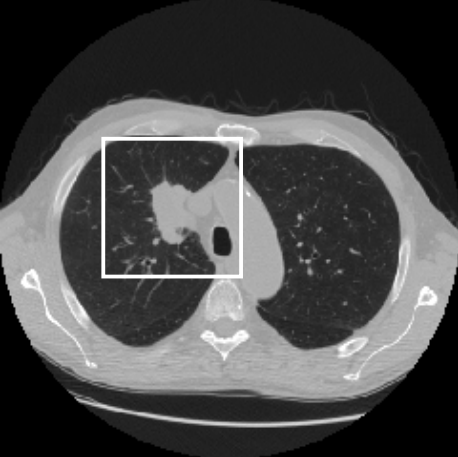
About
Interfaces
This algorithm implements all of the following input-output combinations:
Model Facts
Summary
This algorithm analyzes non-contrast CT scans of the thorax and predicts the lung cancer risk. The algorithm is described in this publication by Fangzhou Liao, Ming Liang, Zhe Li, and Xiaolin Hu and the code is publicly available on Github.
This algorithm was developed as part of the Kaggle Data Science Bowl in 2017 and won the first place in this challenge.
Image courtesy of Fangzhou Liao et al. in the previously mentioned paper.
Mechanism
The algorithm is described in this publication by Fangzhou Liao, Ming Liang, Zhe Li, and Xiaolin Hu.
Validation and Performance
Uses and Directions
This algorithm was developed for research purposes only.
Warnings
Common Error Messages
Information on this algorithm has been provided by the Algorithm Editors, following the Model Facts labels guidelines from Sendak, M.P., Gao, M., Brajer, N. et al. Presenting machine learning model information to clinical end users with model facts labels. npj Digit. Med. 3, 41 (2020). 10.1038/s41746-020-0253-3