Fluid Segmentation in Retinal Optical Coherence Tomography (OCT)
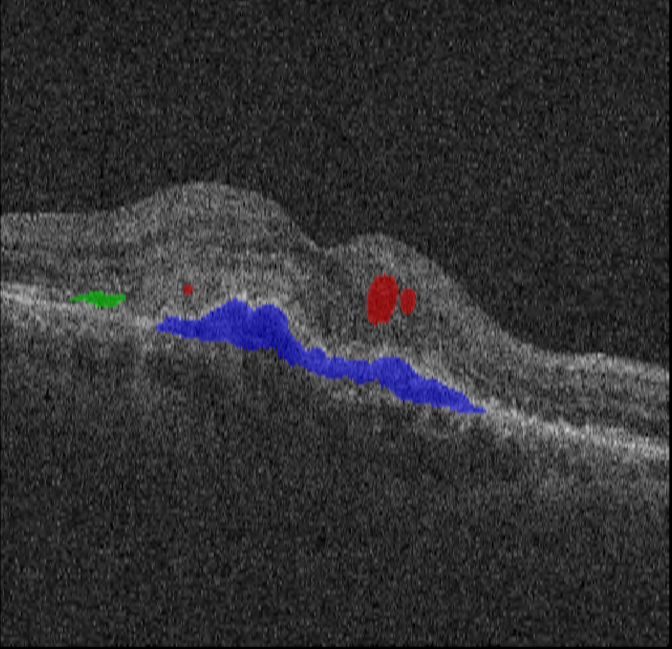
About
- Generic Medical Image
- Generic Overlay (An overlay of unknown type. Legacy, please use alternative interfaces.)
- Results JSON File (A collection of results of unknown type. Legacy, if possible please use alternative interfaces.)
Model Facts
Summary
This algorithm segments intraretinal fluid, subretinal fluid, and pigment epithelial detachments in OCT scans. Optimized for Spectralis, Cirrus and Topcon scanners. The data was developed with training data from the RETOUCH challenge.
This algorithm was developed by the Diagnostic Image Analysis Group and Amsterdam University Medical Center. It is currently maintained by Coen de Vente.
Mechanism
💾 Input and output
Users can upload their 3D OCT images and inspect the results using a web-based image viewer or directly download the segmentation masks. In the output masks, each fluid will be assigned a different scalar value:
- Intraretinal Fluid (IRF)
- Subretinal Fluid (SRF)
- Pigment Epithelium Detachments (PED)
Note, the algorithm works on full OCT scans, not on individual B-scans. For further input requirements, see the image upload page.
🕹 Algorithm description
The algorithm is the 3D variant of nnU-Net.
Validation and Performance
Dice scores (std. dev.)¶
IRF | SRF | PED | |
---|---|---|---|
Cirrus | 0.79 (0.07) | 0.71 (0.27) | 0.61 (0.31) |
Spectralis | 0.76 (0.07) | 0.64 (0.28) | 0.73 (0.25) |
Topcon | 0.54 (0.29) | 0.84 (0.08) | 0.76 (0.13) |
Uses and Directions
This algorithm was developed for research purposes only.
Warnings
Common Error Messages
Information on this algorithm has been provided by the Algorithm Editors, following the Model Facts labels guidelines from Sendak, M.P., Gao, M., Brajer, N. et al. Presenting machine learning model information to clinical end users with model facts labels. npj Digit. Med. 3, 41 (2020). 10.1038/s41746-020-0253-3