HookNet-Breast
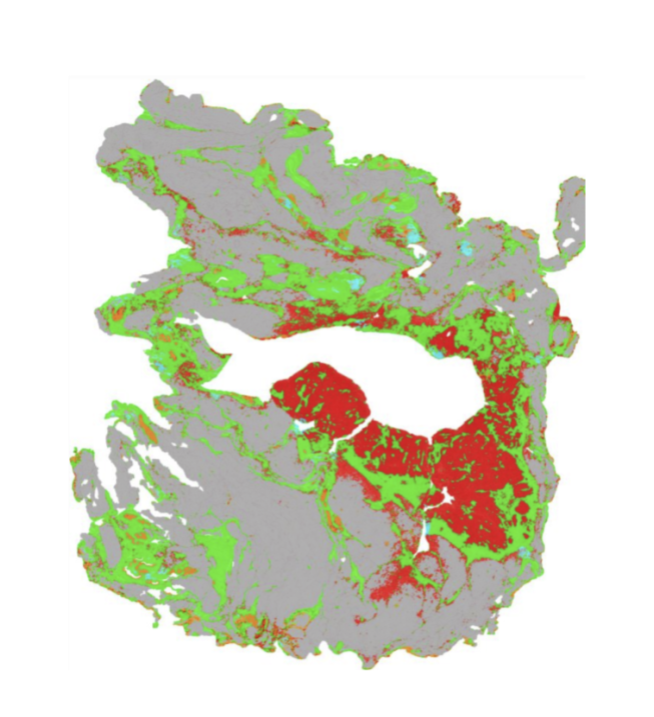
About
- Generic Medical Image
- Generic Overlay (An overlay of unknown type. Legacy, please use alternative interfaces.)
- Generic Overlay (An overlay of unknown type. Legacy, please use alternative interfaces.)
Model Facts
Summary
HookNet algorithm for the segmentation of histopathology breast tissue including ductal carcinoma in situ, invasive ductal carcinoma, invasive lobular carcinoma, non-malignant epithelium, fat, and other breast tissue.
HookNet is a multi-resolution semantic segmentation deep learning model that combines context and details via encoder-decoder branches. Results show that HookNet can simultaneously deal with subtle differences at high-resolution and contextual information. Furthermore, it has the potential to be helpful for any application where context and details are essential.
Requirements¶
Please take the following limitations into account when submitting data to this algorithm: All input images should be in .tif format and contain magnification levels that correspond to a 0.5, and 8.0μm pixel spacing (± 0.25). Furthermore, a tissue mask at 0.5μm pixel spacing (± 0.25) should be provided as well.
paper: https://www.sciencedirect.com/science/article/pii/S1361841520302541
code: https://github.com/DIAGNijmegen/pathology-hooknet
Mechanism
This algorithm has been developed for histopathology H&E stained breast slides. The algorithm expects two inputs: 1) the H&E slide, 2) a tissue-segmentation mask (which can be obtained via this algorithm (https://grand-challenge.org/algorithms/tissue-background-segmenation/))
Validation and Performance
Uses and Directions
This algorithm was developed for research purposes only.
Warnings
Common Error Messages
Information on this algorithm has been provided by the Algorithm Editors, following the Model Facts labels guidelines from Sendak, M.P., Gao, M., Brajer, N. et al. Presenting machine learning model information to clinical end users with model facts labels. npj Digit. Med. 3, 41 (2020). 10.1038/s41746-020-0253-3