HookNet-TLS
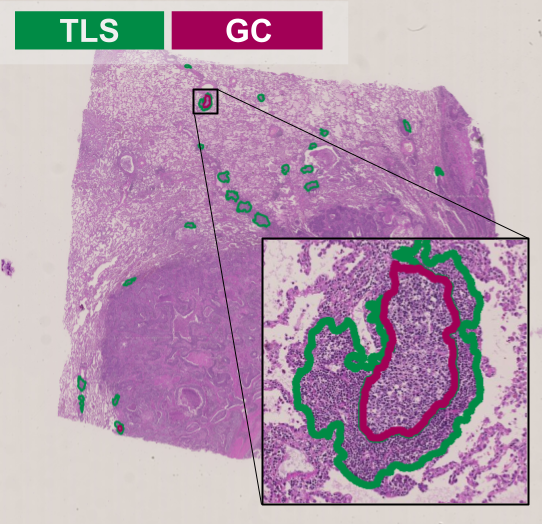
About
- Generic Medical Image
- Generic Overlay (An overlay of unknown type. Legacy, please use alternative interfaces.)
- Tertiary lymphoid structures and Germinal Centers Segmentation (A segmentation mask including Tertiary lymphoid structures (TLSs, voxel value 1) and Germinal Centers (GCs, voxel value 2), Background has voxel value 0).)
- Tertiary lymphoid structures and germinal centers polygons (Polygons of tertiary lymphoid structures and germinal centers)
Model Facts
Summary
HookNet-TLS is a powerful deep-learning algorithm designed for the automated detection of tertiary lymphoid structures (TLS) and germinal centers (GC). The model has been trained on a large set of histology slides from various solid tumors, including clear cell renal cell carcinoma, muscle-invasive bladder cancer, and lung squamous cell carcinoma.
Mechanism
We developed HookNet-TLS, a multi-resolution deep learning model. This model automates the process of TLS quantification and identification of germinal centers in hematoxylin and eosin (H&E) stained digital pathology slides. HookNet-TLS integrates information from multiple image resolutions, mimicking the process a pathologist would use to identify these structures under a microscope. It was developed using 1019 manually annotated slides from The Cancer Genome Atlas (TCGA), encompassing various cancer types. The model's multi-resolution approach allows for a comprehensive analysis of the tissue slides, capturing both the macroscopic and microscopic details necessary for accurate TLS identification.
To use this algorithm, you will need to provide a multiresolution TIFF file that contains 0.5um and 2.0um spacings. If your image is in a different format (e.g. MRXS, SVS, NDPI, etc..), you can use the wholeslidedata package and pyvips to convert it.
Furthermore, as a second input, a tissue background segmentation is required. These can be made with the Tissue-Background Segmentation algorithm
Validation and Performance
Please refer to our paper: Multi-resolution deep learning characterizes tertiary lymphoid structures in solid tumors for validation and performance analysis
Uses and Directions
This algorithm was developed for research purposes only.
Warnings
No warnings are currently applicable.
Common Error Messages
No common error messages are currently known.
Information on this algorithm has been provided by the Algorithm Editors, following the Model Facts labels guidelines from Sendak, M.P., Gao, M., Brajer, N. et al. Presenting machine learning model information to clinical end users with model facts labels. npj Digit. Med. 3, 41 (2020). 10.1038/s41746-020-0253-3