LesionTracer
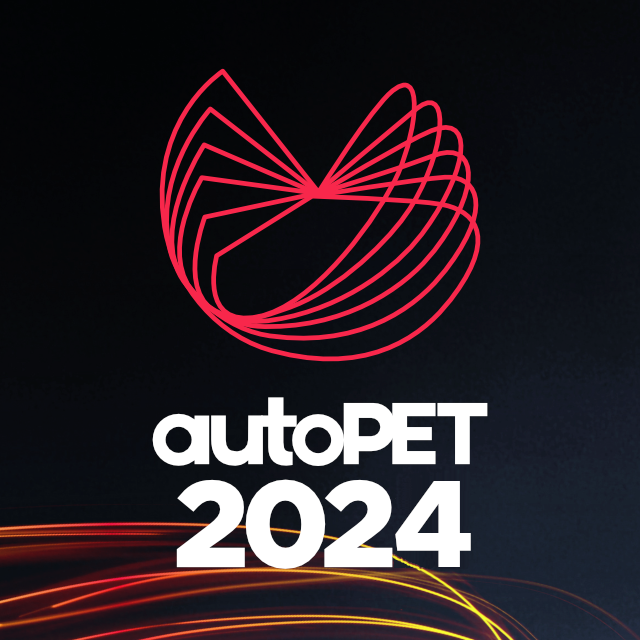
About
Interfaces
This algorithm implements all of the following input-output combinations:
Challenge Performance
Date | Challenge | Phase | Rank |
---|---|---|---|
Sept. 14, 2024 | AutoPET-III | Preliminary Test Set | 12 |
Model Facts
Summary
[autoPET III Winning Solution]
MICCAI 2024 Challenge Submission: Team LesionTracer
https://github.com/MIC-DKFZ/autopet-3-submission
Mechanism
Automated lesion segmentation in PET/CT scans is crucial for improving clinical workflows and advancing cancer diagnostics. However, the task is challenging due to physiological variability, different tracers used in PET imaging, and diverse imaging protocols across medical centers. To address this, the autoPET series was created to challenge researchers to develop algorithms that generalize across diverse PET/CT environments. This paper presents our solution for the autoPET III challenge, targeting multitracer, multicenter generalization using the nnU-Net framework with the ResEncL architecture. Key techniques include misalignment data augmentation and multi-modal pretraining across CT, MR, and PET datasets to provide an initial anatomical understanding. We incorporate organ supervision as a multitask approach, enabling the model to distinguish between physiological uptake and tracer-specific patterns, which is particularly beneficial in cases where no lesions are present. Compared to the default nnU-Net, which achieved a Dice score of 57.61, or the larger ResEncL (65.31) our model significantly improved performance with a Dice score of 68.40, alongside a reduction in false positive (FPvol: 7.82) and false negative (FNvol: 10.35) volumes. These results underscore the effectiveness of combining advanced network design, augmentation, pretraining, and multitask learning for PET/CT lesion segmentation.
Paper: https://arxiv.org/abs/2409.09478
Validation and Performance
Uses and Directions
This algorithm was developed for research purposes only.
Warnings
Common Error Messages
Information on this algorithm has been provided by the Algorithm Editors, following the Model Facts labels guidelines from Sendak, M.P., Gao, M., Brajer, N. et al. Presenting machine learning model information to clinical end users with model facts labels. npj Digit. Med. 3, 41 (2020). 10.1038/s41746-020-0253-3