Lung Cancer Segmentation
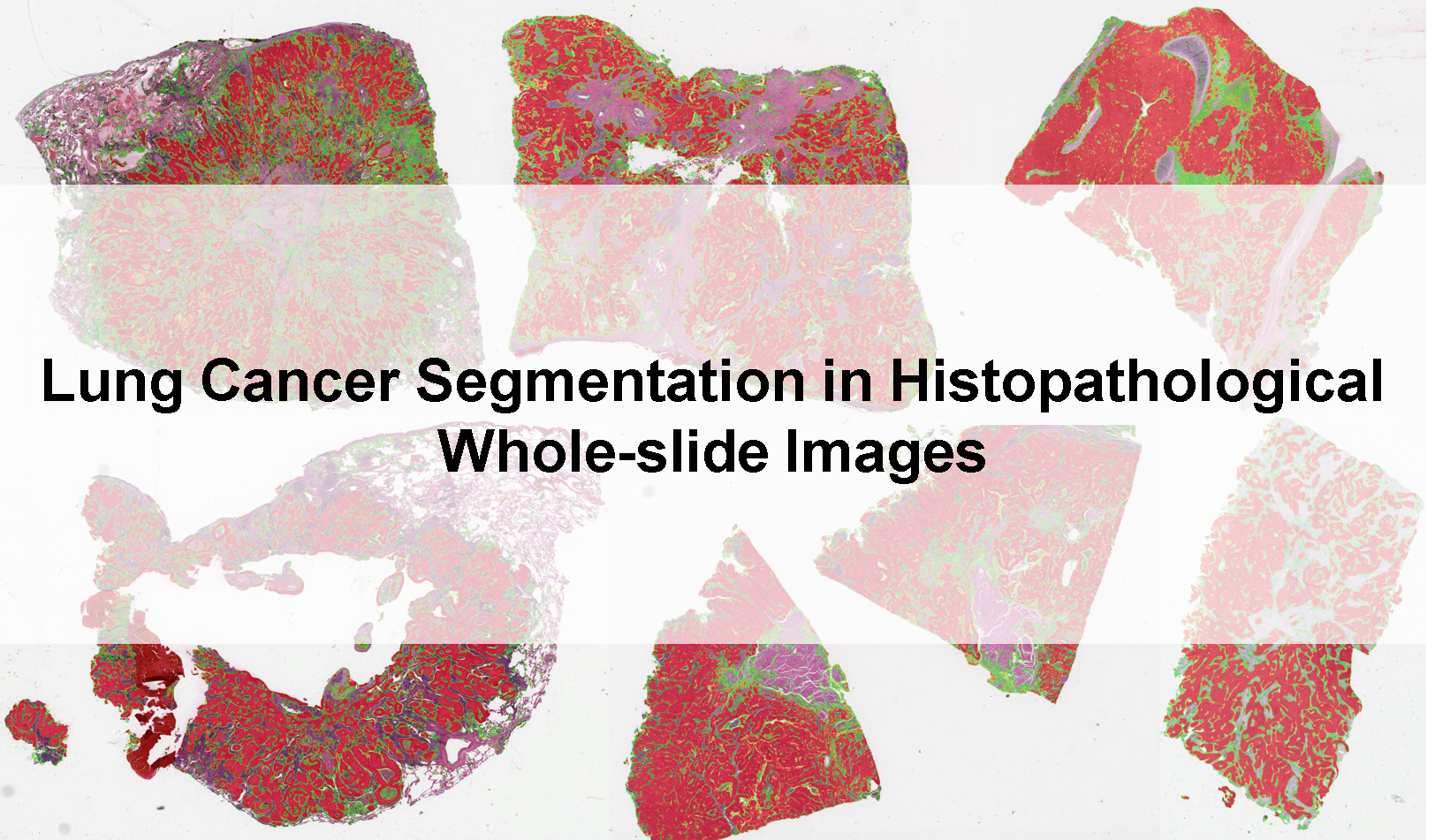
About
Interfaces
This algorithm implements all of the following input-output combinations:
Model Facts
Summary
The model was trained on ACDC-HP challenge and got 3rd place in post-challenge leaderboard. It was further fine-tuned with data from the TCGA-LUAD and TCGA-LUSC projects.
The input images should be whole-slide images, with formats including 'svs', 'tif' etc. The images should have three channels (RGB) and a pixel spacing of about 0.5μm/pixel.
Mechanism
The model is based on U-Net, and trained with data from ACDC-HP challenge, as well as in-house annotations of TCGA-LUAD and TCGA-LUSC
Validation and Performance
Uses and Directions
This algorithm was developed for research purposes only.
Warnings
Common Error Messages
Information on this algorithm has been provided by the Algorithm Editors, following the Model Facts labels guidelines from Sendak, M.P., Gao, M., Brajer, N. et al. Presenting machine learning model information to clinical end users with model facts labels. npj Digit. Med. 3, 41 (2020). 10.1038/s41746-020-0253-3