Lymphocytes detection in immunohistochemistry
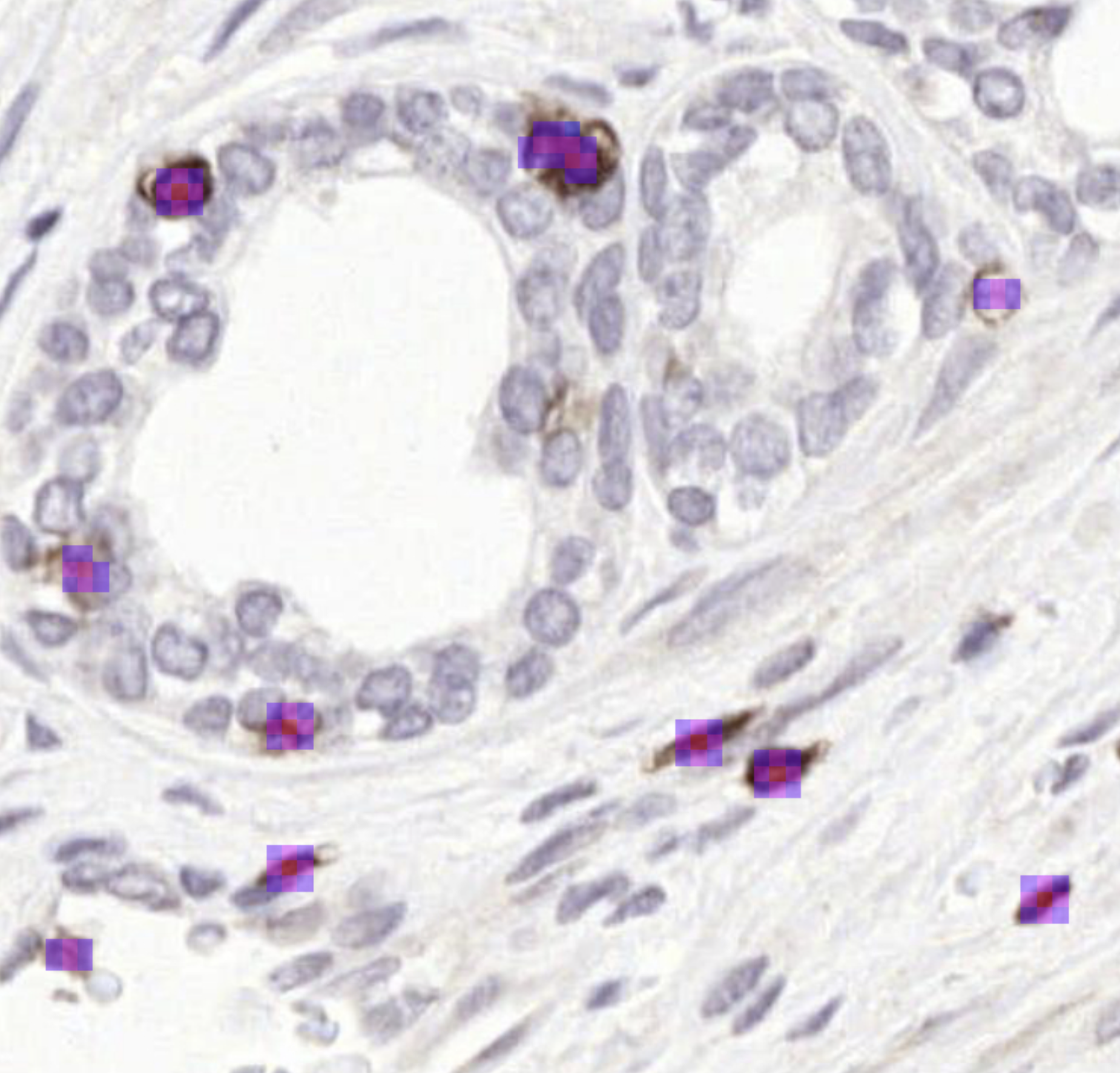
About
- Generic Medical Image
- Generic Overlay (An overlay of unknown type. Legacy, please use alternative interfaces.)
Model Facts
Summary
Clinical problem
The tumor microenvironment consists of a collection of different immune cells that congregate around cancer cells. The lymphocytes influence tumor progression by either inducing cell death or inhibiting immune response. It is hypothesized that they could be used as a biomarker to gauge prognosis and survival in patiënt undergoing immunotherapy.
Quantifying the amount of lymphocytes in the environment of a tumor is a time consuming task which needs to be done by a trained pathologist and is subject to a lot of inter and intra observer variability. There is no standardized methodology to recognize spatial patterns or interactions between lymphocytes by hand. If automated, research into tumor infiltrating lymphocytes could be done much more quickly and consistently.
Solution
The goal of this project was to generalize an existing model that was able to detect lymphocytes in CD3 and CD8 stained slides of prostate cancer, to other types of immunohistochemical staining.
Tissue samples on slides need to be stained before they can be histologically examined using light microscopy and digitized. There is a large variety of immunohistochemical stains to differentiate different lymphocytes by membrane proteins as well as scanners to digitize slides. The ideal model would be able to differentiate lymphocytes in slides of all types of immunohistochemical staining and scanning.
Data
The data consisted of 15 whole slide images (WSI), of slides containing prostate tissue stained with antibodies for CD3, CD8 or ki-67. Slides were obtained and stained in different medical centers, which means a wide range of staining protocols and subsequent subtle differences in appearance are represented in the data. Slides were digitized using a Pannoramic 250 Flash II scanner. Trained analysts picked and annotated several regions in the WSI which either contain regular lymphocyte distribution, clustered lymphocytes or staining artifacts. These WSI’s were randomly divided into a test set containing 5 CD3, 5 CD8 and 5 ki-67 images and a validation set containing CD3, CD8 and ki-67 images.
Mechanism
First smaller patches were randomly extracted from the regions of interest in the training and validation WSI’s. For the training patches, the following augmentations were used: Flipping, Cutout, compression, randomisation of contras or gamma limit and color augmentations. These patches were then used to train a Unet segmentation model.
To quantify the lymphocytes in the data, inference needs to be performed on the images. During inference, the WSI is divided into tiles which are separately analyzed by the model. Then reassembled to provide a mask containing soft-max predictions. These predictions are then post-processed.
To evaluate the performance of the model, the distance between manually annotated coordinates and the coordinate of the top most pixel of the detected area is measured. This method was used to generate a confusion matrix and FROC analysis.
Validation and Performance
Results
Uses and Directions
This algorithm was developed for research purposes only.
Warnings
Common Error Messages
Information on this algorithm has been provided by the Algorithm Editors, following the Model Facts labels guidelines from Sendak, M.P., Gao, M., Brajer, N. et al. Presenting machine learning model information to clinical end users with model facts labels. npj Digit. Med. 3, 41 (2020). 10.1038/s41746-020-0253-3