Nuclei detection in immunohistochemistry
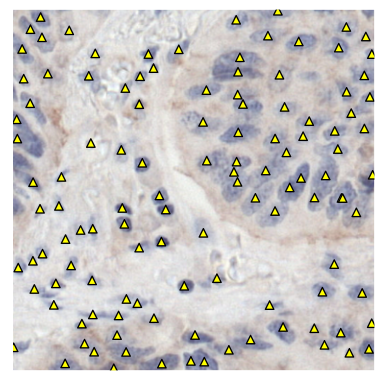
About
- IHC staining for PD-L1
- Tissue Mask (Segmentation of the tissue in the slide. 0: Background 1: Tissue)
- Cell Classification (Predicted cells for cell patches. Contains the coordinates, the class in the 'name' attribute, and the 'probability' reflecting the confidence of each predicted cell.)
- Nuclei segmentation (A segmentation mask that labels (viable) nuclei in immunohistochemistry stains.)
Model Facts
Summary
This algorithm detects viable nuclei stained with hematoxylin in immunohistochemistry (IHC) stained whole-slide images. This algorithm is referred to as a 'nuclei detector' and was developed for the use case of semi-automatic annotation as described in Van Eekelen et al. 2024, but can be used for any theoretical downstream task that first requires the localization of nuclei in IHC.
The nuclei detector was developed by the Computational Pathology research group of the Radboudumc, Nijmegen, The Netherlands, in 2021-2024.
Mechanism
This fully-automatic deep learning based model is meant to detect all nuclei within a IHC stained whole-slide image. It is applied to a WSI in a tile-by-tile fashion, only in places where tissue resides on the WSI (as determined by a tissue background segmentation preprocessing step). For additional methodological details, we refer the user to our paper, linked above.
Validation and Performance
The nuclei detector was validated against four human readers (trained lab asssistants). The four annotators each exhaustively annotated regions of interests (ROIs) for nuclei; the ROIs were selected within non-small cell lung cancer resections taken from the Radboud University Medical Center's archive. In total 12 cases were annotated with 3 regions of interests on average per case, for an average of ~10.000 annotations per reader. The agreement amongst the readers themselves and the readers versus the algorithm was determined via pairwise F1 score, shown below in subfigure A. We define a hit as two paired annotations being 8 microns or closer to each other.
Subfigure A shows that average reader-reader F1 score and average algorithm-reader F1 scores are identical at 0.87, indicating that the nuclei detector performs at the same level as human readers.
Uses and Directions
This algorithm is intended to be used in a research setting only; it is not a diagnostic for cancer nor is it meant to guide or drive clinical care. The terms of usage for Grand Challenge apply to the uploaded slides. To use the algorithm, users need to request access, and only requests from verified users will be accepted. We refer the user to the discussion of our paper (linked above) for details on the performance and limitations of the algorithm.
Warnings
Common Error Messages
Information on this algorithm has been provided by the Algorithm Editors, following the Model Facts labels guidelines from Sendak, M.P., Gao, M., Brajer, N. et al. Presenting machine learning model information to clinical end users with model facts labels. npj Digit. Med. 3, 41 (2020). 10.1038/s41746-020-0253-3