Clinically Significant Prostate Cancer Detection in bpMRI
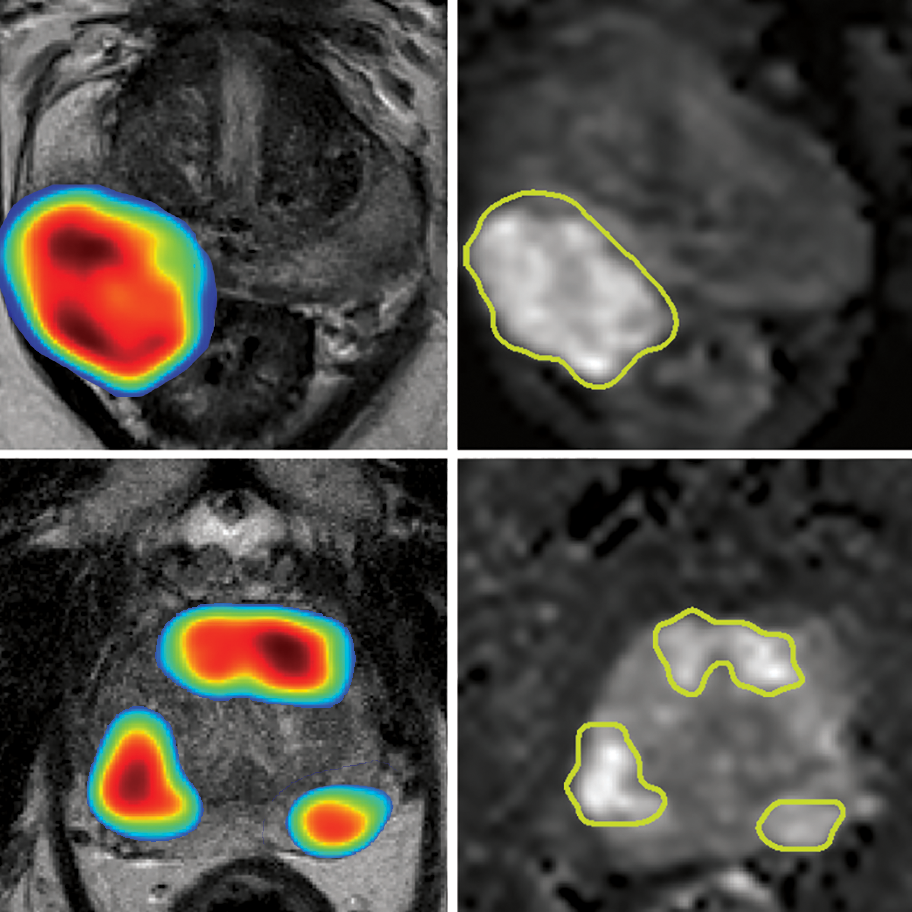
About
- Transverse T2 Prostate MRI (Transverse T2 MRI of the Prostate)
- Transverse HBV Prostate MRI (Transverse High B-Value Prostate MRI)
- Transverse ADC Prostate MRI (Transverse Apparent Diffusion Coefficient Prostate MRI)
- Transverse Cancer Heatmap Prostate MRI (Single-class, probabilistic segmentation of prostate cancer in 3D, where each voxel represents a floating point in range [0,1].)
Model Facts
Summary
Mechanism
This algorithm is a deep learning-based detection/diagnosis model (deep attentive 3D U-Net), which was trained, validated and tested using 2732 prostate biparametric MRI (bpMRI) scans paired with PI-RADS v2 annotations. Its input requires 3 axial/transverse MRI volumes in .mha
format:
- T2-weighted imaging (T2W)
- High b-value diffusion-weighted imaging (HBV)
- Apparent diffusion coefficient map (ADC)
In return, the model predicts a single heatmap for the likelihood of clinically significant prostate cancer, with the same physical dimensions and the same spatial resolution as the input T2W image.
Source Code: https://github.com/DIAGNijmegen/prostateMR_3D-CAD-csPCa/
Related Publications:
Validation and Performance
Uses and Directions
-
For research use only. This algorithm is intended for research purposes only. It was not designed to be a stand-alone diagnostic for prostate cancer, or to be directly applied in clinical routine.
-
Benefits: Risk stratification for clinically significant prostate cancer using prostate MRI is instrumental to reduce over-treatment and unnecessary biopsies among patients.
-
Target population: This algorithm was trained on patients with raised PSA levels or clinical suspicion of prostate cancer, without prior treatment (e.g. radiotherapy, transurethral resection of the prostate (TURP), transurethral ultrasound ablation (TULSA), cryoablation, etc.), without prior biopsies, without artifacts and/or with reasonably-well aligned sequences. It is not suitable for bpMRI scans of patients, outside this demographic.
-
MRI scanner: This algorithm was trained and evaluated exclusively on prostate bpMRI scans derived from 3T Siemens Healthineers (Prisma/Skyra) MRI scanners with surface coils. It does not account for vendor-neutral properties or domain adaptation, and in turn, is not compatible with scans derived using any other MRI scanner or those using endorectal coils.
-
Sequence alignment and position of the prostate: While the input images (T2W, HBV, ADC) can be of different spatial resolutions, the algorithm assumes that they are co-registered or aligned reasonably well and that the prostate gland is localized within a volume of 330 cm³ from the centre coordinate.
-
Before using this model: Test the model retrospectively and prospectively on a diagnostic cohort that reflects the target population that the model will be used upon, to confirm the validity of the model within a local setting.
Warnings
-
Risks: Even if used appropriately, clinicians using this model can misdiagnose cancer. Delays in cancer diagnosis can lead to metastasis and mortality. Patients who are incorrectly treated for cancer can be exposed to risks associated with unnecessary interventions and treatment costs related to follow-ups.
-
Generalizability: This model was not trained on MRI examinations of patients with prior treatment (e.g. radiotherapy, transurethral resection of the prostate (TURP), transurethral ultrasound ablation (TULSA), cryoablation, etc.), prior positive biopsies, artefacts or misalignment between sequences. It was not trained on MRI exams obtained from any other scanner, besides 3T Siemens Healthineers (Prisma/Skyra) scanners with surface coils. Hence it is susceptible to faulty predictions and unintended behaviour when presented with such cases.
-
Clinical rationale: The model is not interpretable and does not provide a rationale for high risk scores, beyond the localization structures presented in its output heatmap. Clinicians are expected to place the model output in context with other clinical information to make the final determination of diagnosis.
-
Discontinue use if: Clinical staff raise concerns about the utility of the model for the intended use-case or large, systematic changes occur at the data level that necessitates re-training of the model.
Common Error Messages
Information on this algorithm has been provided by the Algorithm Editors, following the Model Facts labels guidelines from Sendak, M.P., Gao, M., Brajer, N. et al. Presenting machine learning model information to clinical end users with model facts labels. npj Digit. Med. 3, 41 (2020). 10.1038/s41746-020-0253-3