Scaphoid fracture detection
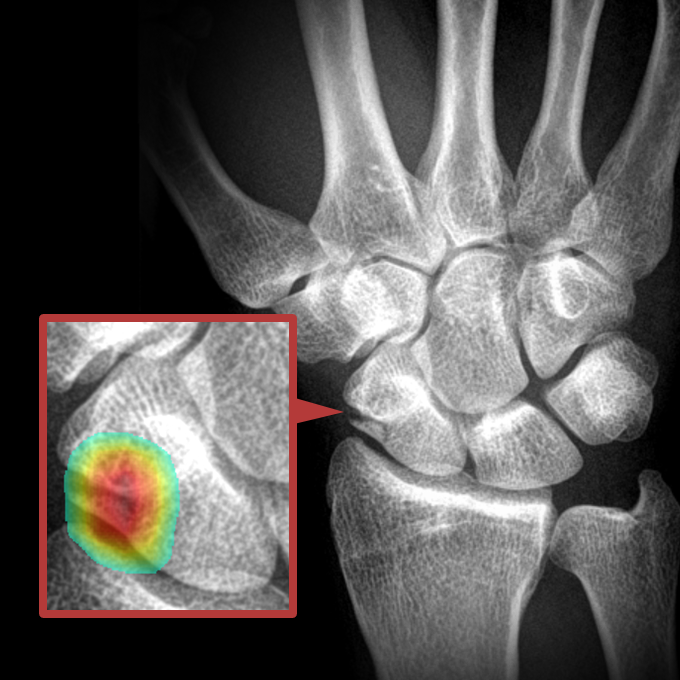
About
- Generic Medical Image
- Generic Overlay (An overlay of unknown type. Legacy, please use alternative interfaces.)
- Results JSON File (A collection of results of unknown type. Legacy, if possible please use alternative interfaces.)
Model Facts
Summary
This model is based on convolutional neural networks and uses conventional radiographs of the hand, wrist, and scaphoid to detect scaphoid fractures. It applies two networks consecutively: a segmentation network localizes the scaphoid and passes the relevant region to a detection network for fracture detection. It was developed in 2019-2020 at the Radboud University Medical Center and Jeroen Bosch Hospital in the Netherlands. Only radiographs with an anterior-posterior or a posterior-anterior projection of the hand in a neutral position are supported. The validation results of the model were published in Hendrix et al (2021).
This algorithm description is adapted from the template proposed by Sendak, Gao, Brajer, and Balu (2020).
Mechanism
Input:
- Data type: anterior-posterior (AP) or posterior-anterior (PA) radiograph (x-ray) of the hand, wrist, or scaphoid.
- File format: DICOM or MHA file containing the Pixel Spacing Attribute (tag: 0028,0030) or Imager Pixel Spacing Attribute (tag: 0018,1164). The Photometric Interpretation Attribute (tag: 0028,0004) should be "MONOCHROME2" (minimum value is intended to be displayed as black).
- Target population: all patients with a sufficiently developed scaphoid bone (at least seven years old, preferably older than 18 years).
Output:
- Score: scaphoid fracture score between 0 (fracture absent) and 1 (fracture present).
- Overlay: class activation map with a heat map color coding that indicates which regions in the radiograph were influential to the prediction of the model: colder (blue-green) and warmer (yellow-red) colors respectively indicate low and high importance regions. The red canvas represents the selected region provided to the fracture detection network.
Model type: Convolutional neural network.
Training data location and time-period: Picture archiving and communication systems of the Radboud University Medical Center and Jeroen Bosch Hospital in the Netherlands, radiographs acquired between 2003 - 2020.
Validation and Performance
Scaphoid segmentation network:
- Evaluation metrics: Dice similarity coefficient (DSC) and (symmetric) Hausdorff distance (HD).
- Ground truth: manually drawn segmentation mask of the scaphoid.
- Test set: 208 hand, wrist, and scaphoid radiographs from the Radboud University Medical Center and Jeroen Bosch Hospital.
- Results: the network achieved an average DSC of 97.4% ± 1.4 with an HD of 1.31 mm ± 1.03.
Scaphoid fracture detection network:
- Evaluation metrics: sensitivity, specificity, positive predictive value (PPV), and area under the receiver operating characteristic curve (AUC). Automated image crops were used for evaluation.
- Ground truth: binary scaphoid fracture label (fracture present/absent) obtained using a CT scan following conventional radiographs of the hand, wrist, or scaphoid within four weeks.
- Test set: 190 hand, wrist, and scaphoid radiographs (95 fracture, 95 no fracture) from the Jeroen Bosch Hospital.
- Results: the network achieved a sensitivity of 78% (95% CI: 70, 86), specificity of 84% (95% CI: 77, 92), PPV of 83% (95% CI: 77, 90), and AUC of 0.87 (95% CI: 0.81, 0.91).
Uses and Directions
Benefits: Automated scaphoid fracture diagnosis may diminish the risk of missing a fracture, reduce the costs of additional imaging studies and unnecessary therapy, speed up diagnosis, and allow earlier treatment.
Target population and use case: Patients clinically suspected of having a scaphoid fracture typically undergo conventional radiography. The model preprocesses incoming wrist, hand, and scaphoid radiographs in the picture archiving and communication system, and may be able to assist residents, radiologists, or other physicians by acting either as a first or second reader, or as a triage tool that helps prioritizing worklists.
General use: This model is intended to be used by radiologists for identifying scaphoid fractures on conventional radiographs of the hand, wrist, or scaphoid. It is not a diagnostic for scaphoid fractures and is not meant to guide or drive clinical care. It should only be used to complement other pieces of patient information related to scaphoid fractures as well as a physical evaluation to determine the need for scaphoid fracture treatment.
Appropriate decision support: The model predicts whether any scaphoid fractures are present. The radiologist examines the predicted scaphoid fracture score and provided visual evidence along with other clinical information to determine if a scaphoid fracture is present.
Before using this model: Test the model retrospectively on a diagnostic cohort that reflects the target population that the model will be used upon to confirm validity of the model within a local setting.
Safety and efficacy evaluation: An observer study with retrospectively collected data was conducted and showed that the model was able to detect scaphoid fractures just as well as 11 radiologists, achieving a comparable AUC. Future research should investigate to what extent this model could improve the diagnostic performance of radiologists.
Warnings
Risks: Even if used appropriately, radiologists using this model can misdiagnose scaphoid fractures. Delays in diagnosing a scaphoid fracture put patients at a greater risk of developing a non-union fracture, which may lead to complications such as avascular necrosis, carpal instability, osteoarthritis, and ultimately functional loss. False positive diagnoses lead to unnecessary wrist immobilization, which increases health expenditure and decreases patients’ productivity.
Inappropriate settings: This model was not trained or evaluated on radiographs in which the scaphoid is incompletely depicted, obstructed by casts or implants, excessively damaged or malformed, (partially) resected, underdeveloped (children), or not depicted with an AP or a PA projection. Do not use the model when any of these circumstances apply. Only use radiographs that have been made specifically for fracture diagnosis and depict a hand or wrist in a neutral position (i.e. fingers pointing upwards).
Clinical rationale: The model provides visual evidence for the predicted scaphoid fracture probability. This evidence is only interpretable as long as the input radiograph is similar to the radiographs used for training the model. Clinical end users are expected to place the model output in context with other clinical information to make final determination of diagnosis.
Inappropriate decision support: This model may not be accurate outside of the target population (primarily adult patients). This model is not a diagnostic and is not designed to guide clinical diagnosis and treatment for scaphoid fractures.
Generalizability: This model was evaluated within the local setting of the Radboud University Medical Center and Jeroen Bosch Hospital. Do not use this model in an external setting without further evaluation.
Discontinue use if: Clinical staff raise concerns about the utility of the model for the indicated use case or large, systematic changes occur at the data level that necessitates re-training of the model.
Common Error Messages
"Uneven pixel spacing encountered. Image scaling might not be accurate": The uploaded image has a different pixel spacing in the x and y direction. The model uses the pixel spacing information to rescale the image in order to normalize the scaphoid size before segmentation. Provided that the pixel spacing information is inaccurate, the scaling operation may result into an unnatural looking image.
"Pixel spacing of (1, 1) encountered. Pixel spacing information could be wrong or missing": The uploaded image most likely does not contain valid pixel spacing information. Accurate pixel spacing information is required to normalize the scaphoid size before segmentation.
"Bounding box is relatively small or large. Possible scaphoid segmentation failure": The height or width of the bounding box derived from the generated scaphoid segmentation mask is outside three standard deviations from the mean height or width of the bounding boxes in the training data. This may indicate a failure of the segmentation network to localize the scaphoid.
"Cannot classify the image due to segmentation failure": The segmentation network failed to segment any pixels of the scaphoid and no bounding box could be generated. Therefore, there is no region of interest to process by the fracture detection network.
Information on this algorithm has been provided by the Algorithm Editors, following the Model Facts labels guidelines from Sendak, M.P., Gao, M., Brajer, N. et al. Presenting machine learning model information to clinical end users with model facts labels. npj Digit. Med. 3, 41 (2020). 10.1038/s41746-020-0253-3