Tibia bone and implant segmentation
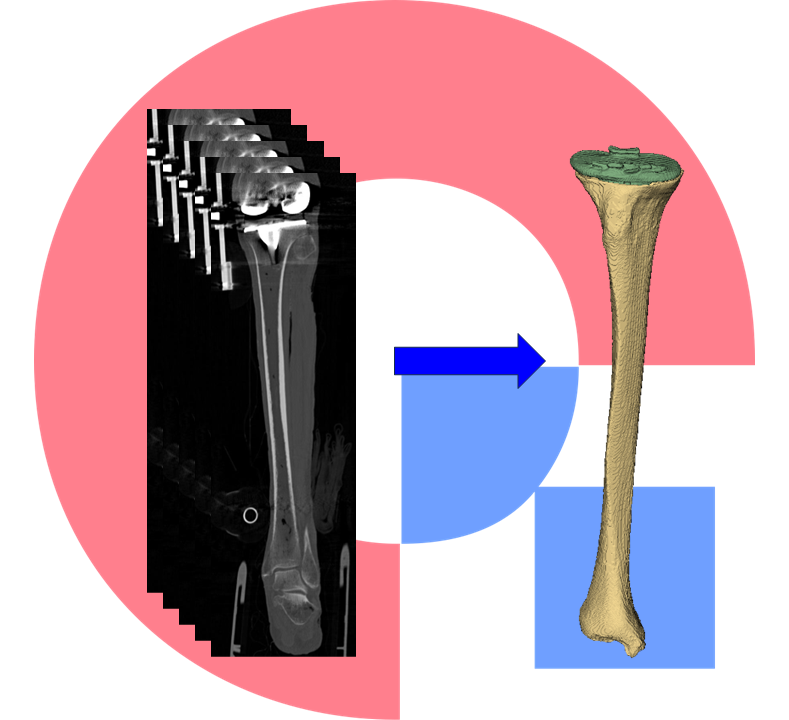
About
- CT Image (Any CT image)
- Tibia implant and bone segmentation - unilateral (3D tibia implant segmentation with class mapping 1: tibia implant, 2: tibia bone)
Model Facts
Summary
This model is based on convolutional neural networks and uses 3D CT scans of knee implants as input. 4 models trained on different dataset partitions are applied and their results are aggregated to an ensemble for the final segmentation mask. The model was developed in 2023-2024 at the Amsterdam UMC, location UvA, in the Netherlands. The first validation results of the model were published in Magg et al. (2024). In the meantime, the model has been updated and retrained with an updated datasets (see current results in Validation and performance section).
Mechanism
Input:
- Data type: 3D CT scan of the lower leg (femur TKA component to ankle joint).
- File format: NIFTI or MHA file
- Target population: patients with a TKA (total knee arthoplasty)
Output:
- Data type: Segmentation with 2 classes:
- the tibial TKA component (voxel value 1)
- the tibial cortex bone (voxel value 2)
- File format: MHA file
Model type: Convolutional neural network
Validation and Performance
- Evaluation metrics: Dice similarity coefficient (DSC) and 95%-percentile Hausdorff distance (HD95).
- Ground truth: manually drawn segmentation mask of the tibia bone and implant component.
- Test set: 4 fold patient-based cross-validation
- Results: the network achieved an average DSC of 95.56% with an HD95 of 0.58 mm.
Uses and Directions
This algorithm was developed for research purposes only.
Warnings
Do not use this model in an external setting without further evaluation.
Common Error Messages
Information on this algorithm has been provided by the Algorithm Editors, following the Model Facts labels guidelines from Sendak, M.P., Gao, M., Brajer, N. et al. Presenting machine learning model information to clinical end users with model facts labels. npj Digit. Med. 3, 41 (2020). 10.1038/s41746-020-0253-3