Tumor proportion score in non-small cell lung cancer
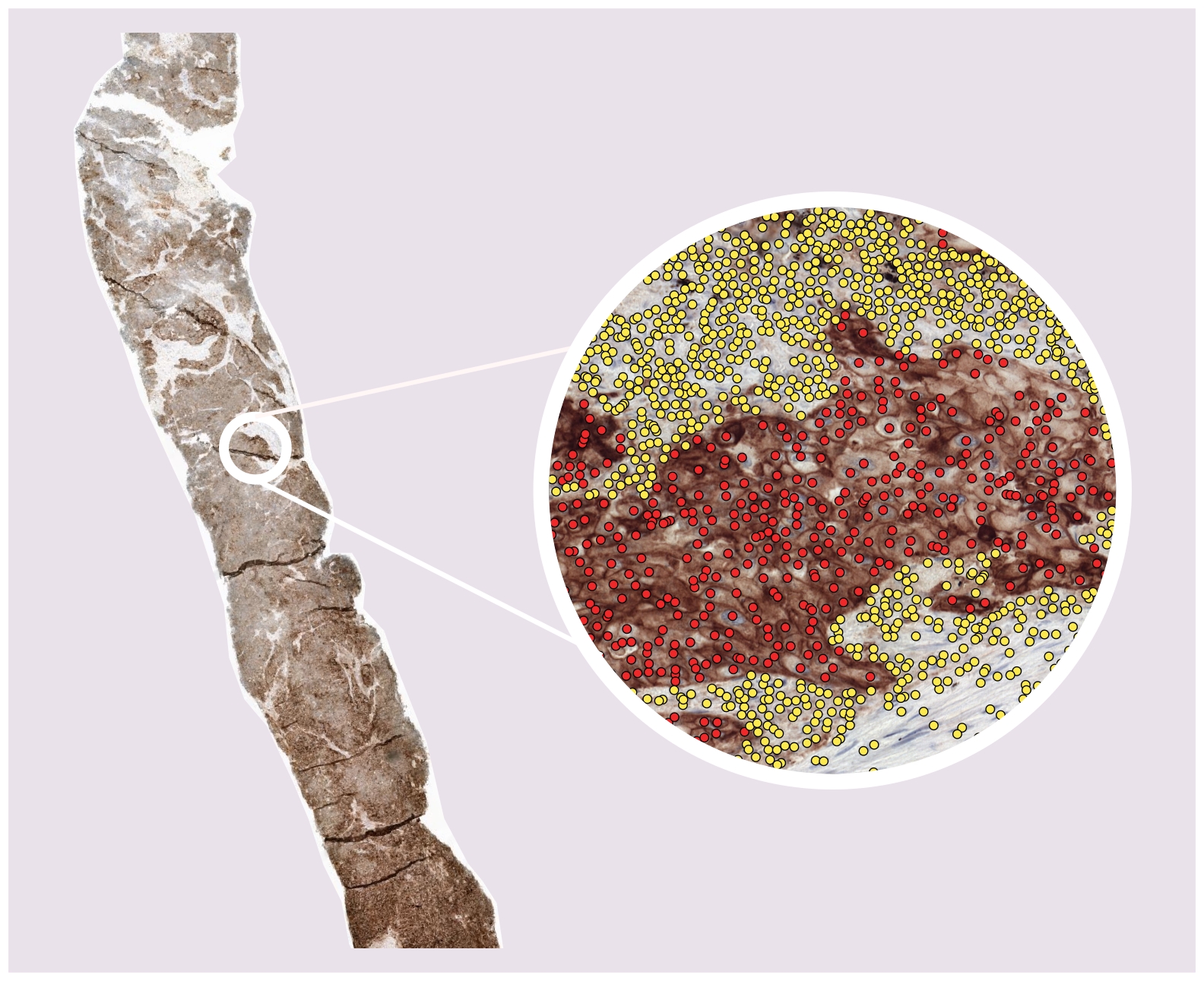
About
Interfaces
This algorithm implements all of the following input-output combinations:
Inputs | Outputs | |
---|---|---|
1 |
Model Facts
Summary
This algorithm uses a PD-L1 immunohistochemistry (IHC) stained whole-slide image (WSIs) to predict the tumor proportion score (TPS): the percentage of (viable) PD-L1 positive tumor cells as compared to the total number of (viable) tumor cells in the WSI. It does so by localizing and classifying cells within the WSI as PD-L1 negative or positive tumor cells ( or 'other cells', which are not taken into account for calculating the TPS). Both the TPS and the localized cells are given as output by the algorithm.
The algorithm is referred to as a 'PD-L1 detector' and was developed by the Computational Pathology research group of the Radboudumc, Nijmegen, The Netherlands, in 2021-2024.
Mechanism
This is a fully-automatic deep learning based model for determining the TPS via the localization and classification of cells in PD-L1 stained IHC of NSCLC patients. The model is applied to the WSI in a tile-by-tile fashion, only in places where tissue resides on the WSI (as determined by a tissue background segmentation). When all tiles of the WSI have been processed, the TPS is calculated by counting the number of PD-L1 positive and negative tumor cell detections and calculating: #pos / (#pos + #neg).
The model was developed using a semi-automated annotation strategy where homogeneous tissue regions (e.g. all PD-L1 positive tumor cells, all stroma, etc) were labelled. An auxiliary nuclei detector (trained separately, available here) was used to detect the individual nuclei within the regions. The final PD-L1 detector was trained on the detected nuclei intersected with the labeled regions. In total, we train on a diverse set of 29 cases from two different centers, three different PD-L1 stains and 415.764 point annotations.
For additional details, we refer the user to our paper, linked above.
Validation and Performance
The PD-L1 detector was evaluated on two aspects.
- Agreement between the algorithm and 6 expert pathologists on cell-level prediction of PD-L1 expression (i.e. positive or negative tumor cells)
- Agreement between the algorithm and 6 expert pathologists on slide-level TPS
For the cell-level prediction of PD-L1 expression, we invited expert pathologists to make manual annotation within regions-of-interest over a large and diverse case set of multiple NSCLC subtypes, PD-L1 monoclones and clinical centers. We evaluated their agreement amongst each other and with the algorithm via pairwise F1 score; the algorithm performs within inter-rater variability on in-domain cases (RUMC, NEG) but struggles slightly on out-of-domain cases (NKI).
For determining the slide-level TPS, we invited expert pathologists to visually estimate TPS in 5% increments in a diverse set of a hundred cases. We measured Cohen's kappa as an agreement metric over the clinically relevant cutoffs (>1%, >=50%) and find that for the difficult 1% cutoff, our PD-L1 detector has a better agreement with the majority vote than four out of six readers.
Uses and Directions
This algorithm is intended to be used in a research setting only; it is not a diagnostic for cancer nor is it meant to guide or drive clinical care. The terms of usage for Grand Challenge apply to the uploaded slides. To use the algorithm, users need to request access, and only requests from verified users will be accepted. We refer the user to the discussion of our paper (linked above) for details on the performance and limitations of the algorithm.
Warnings
Common Error Messages
Information on this algorithm has been provided by the Algorithm Editors, following the Model Facts labels guidelines from Sendak, M.P., Gao, M., Brajer, N. et al. Presenting machine learning model information to clinical end users with model facts labels. npj Digit. Med. 3, 41 (2020). 10.1038/s41746-020-0253-3