Spring Newsletter 2022
Published 11 April 2022
🛠️ New features of Grand Challenge¶
We kicked this year off by refining our user documentation of Grand Challenge. You can access it through the ‘Help’ button in the top navigation bar or go there directly via this link. The documentation contains information about how to get started on Grand Challenge as well as detailed guides for how to participate in and host your own Challenges, Algorithms, Archives and Reader Studies and it also includes sample scripts for the Grand Challenge API client.
💡 Blogposts¶
“Can AI predict breast cancer recurrence via automated quantification of tumour-infiltrating lymphocytes?”¶
The "TIGER" challenge, which will run until the end of April 2022, is the first challenge that aims to fully automate the assessment of tumour-infiltrating lymphocytes in H&E slides of breast biopsies. Together with the participants, the organizers of the TIGER challenge aim to find the best AI-based solutions for the assessment of the TILs and production of a “TILs score” that predicts the progression and therapy responsiveness of breast cancer. In this blogpost, the relevancy and impact of the challenge is summarized together with the future perspectives for the best performing algorithms.
“Learn about AI in healthcare with these online courses”¶
An overview of the current courses that try to bridge the gap between AI and healthcare is presented and explained in detail by Kicky van Leeuwen to get a feeling of which course would suit you best, what time it takes to learn and what the prices are for the offered courses.
🔦 Highlighted algorithms¶
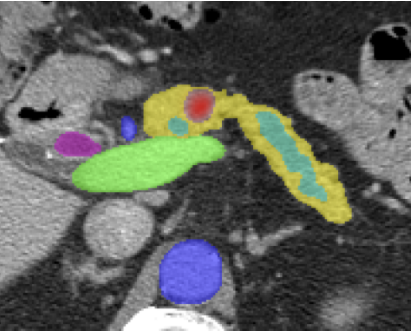
Pancreatic ductal adenocarcinoma detection
This 3D nnU-Net based algorithm produces a tumour likelihood heatmap for the presence of pancreatic ductal adenocarcinoma in portal venous contrast enhanced computed tomography images. Additionally, the algorithm provides the segmentation of multiple surrounding anatomical structures such as pancreatic duct, common bile duct, veins, and arteries. The AUC for the whole test cohort is 0.909. Try out this algorithm with your own data!Prostate cancer detection
This nnU-Net based algorithm predicts a heatmap for the likelihood of clinically significant prostate cancer using bi-parametric magnetic resonance images. The algorithm ensembles fifteen independent models that were trained jointly on manually and report-guided automatically annotated MRI examinations. The AUC of the algorithm is 0.901. Try out this algorithm with your own data!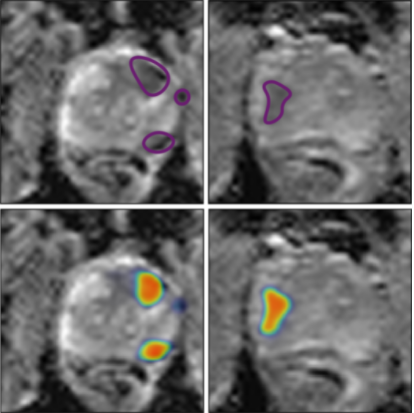
🚀 Upcoming challenges¶
Currently, there are two type of challenges on Grand Challenge. A type 1 challenge is where the training data (including ground truth) and testing data (excluding ground truth) are made publicly available with only the ground truth files for testing data to be kept secret. Participants are expected to upload predictions of the publicly available testing data, and Grand Challenge automatically evaluates the predictions by comparing them with the secret labels. In a type 2 challenge, participants submit an algorithm as a solution. This algorithm is then run on a secret test set (uploaded to an archive by the challenge admins) on the Grand Challenge platform. The results that the algorithm produces are subsequently evaluated using a custom evaluation method provided by the challenge admins. With the introduction of type 2 challenges, most challenges are moving to become type 2 challenges.
🥅 Goal: To benchmark AI against radiologists at prostate cancer detection in MRI, using the largest public training dataset and private testing cohort reported in prostate-AI literature till date
✍️ Register: Accepting early registrations now. Full release of challenge website on 1st April 2022
⏰ Start: "Public Training and Development Phase" of AI algorithms, tentatively begins on 1st May 2022, with the release of the public training dataset
🏆 Prize: To-be-announced
🥅 Goal: To evaluate new computer algorithms for the automated assessment of tumour-infiltrating lymphocytes in Her2 positive and Triple Negative breast biopsy histopathology slides
✍️ Register: Leaderboard 1 was opened on February 4th and leaderboard 2 was opened on March 10th
⏰ Start: February 4th, 2022, and ending on April 20th, 2022
🏆 Prize:
Leaderboard 1 (computer vision performance):
- AWS credits to value of $1.000,-
- AWS credits to value of $1.000,-
- AWS credits to value of $1.000,-
Leaderboard 2 (prognostic value):
- AWS credits to value of $5.000,-
- AWS credits to value of $3.000,-
- AWS credits to value of $2.000,-
🥅 Goal: To segment and label of teeth with a focus on teeth position and shape variation across subjects
✍️ Register: Accepting participation submissions from March 1st, 2022
⏰ Start: March 15th, 2022, is expected release date for training data batch
🏆 Prize: To-be-announced
🥅 Goal: To automatically register breast cancer tissue in whole-slide-images with real-world data since all slides in this challenge originate from routine diagnostic workflows
✍️ Register: Accepting participation submissions
⏰ Start: Release of training data on April 1st, 2022
🏆 Prize: Two sets of monetary challenge prizes will be distributed among the participants. All participants will be eligible to receive prize money from the first set, whereas only those teams that publish their code will be eligible for prize money from the second set.
Additional to these challenges, more challenges can be found here.
🏆 Leadboard of finished challenges¶
The goal is to develop a high-performance open-source solution for the detection and generation of pulmonary nodules on chest radiograph to drive towards early detection of lung cancer. The winners for the detection track are Finn Behrendt (AUC: 0.8390), Radu Miron (AUC: 0.8275) and Di Xu (AUC: 0.8011). Congratulations!
The goal is to develop solutions for glaucoma screening from color fundus photography that are robust to real-world scenarios. The winners of the final test phase, measured by referable glaucoma pAUC, referable glaucoma sensitivity at 95% specificity, ungradability kappa and ungradability AUC, are He Wang (Mean position: 1.8), with Firas Khader and Temirgali Aimyshev having a tie (Mean position: 3). Congratulations!