Autumn Newsletter 2022
Published 1 Nov. 2022
🛠️ New features of Grand Challenge¶
While heading towards the end of 2022, we haven't sat still over the summer holidays and the start of the new academic year! Last quarter, we mainly focused on optimizing our viewers for radiology and pathology images. Specialized views were added to the custom hanging protocols in the last cycle. Now, a ‘3D-side view' and ‘minimap’ allow for a more customizable viewing experience. Moreover, there is now a plane indicator in the CIRRUS viewer that shows the viewport of the current slice (axial, coronal, or sagittal). Additionally, it is now possible for pathology images to rotate by 90° steps. This is especially useful when viewing different stainings of the same tissue side by side, but one of them is scanned in a different direction. To learn more about these viewer upgrades for different modalities, visit the blog posts considering the upgrades for August and September!
💡 Blogposts¶
"When AI meets the TILs: results from the TIGER challenge"¶
TIGER -- the first challenge on fully automated assessment of tumor-infiltrating lymphocytes (TILs) in breast cancer -- was organized by researchers from the Radboud University Medical Center (Netherlands). Sponsored by AWS, TIGER was organized in collaboration with an international network of clinical and academic partners. TIGER ran until April 2022. This blog post summarizes the challenge and includes a discussion of the results, along with the prognostic value of the AI-based TILs.
“STOIC2021 results webinar”¶
The final results for the STOIC2021 are in! Held with a set of 10,000+ CT scans from the STOIC project, participants predicted who will develop severe COVID-19 from a CT scan. STOIC2021 implements a new AI challenge format where participants still developed their solutions with a public set of training data (similar to Type 1 and 2 challenges), but instead of training their final solution themselves, they submitted training code to the challenge organizers who then trained these systems on a very large secret training set.
🔦 Highlighted algorithms¶
Endometrial Carcinoma classification
This model classifies whether (pre)malignant tissue is present in a WSI. It, therefore, only requires a WSI as input and will from this produce both a likelihood of the (pre)malignancy being present and a heatmap, which indicates where in the WSI the tissue should be located. The model outputs the likelihood between 0 and 1 stating the possibility of (pre)malignant tissue being present in the endometrium. The AUC for the test cohort using all 5 folds in an ensemble is 0.960. Try out this algorithm with your own data!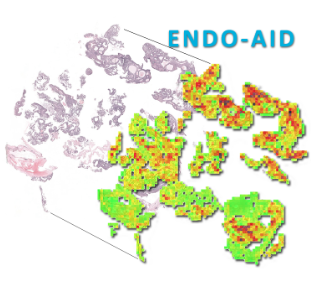

Whole heart segmentation in 3D contrast-enhanced CT
This 3D convolutional neural network (CNN) architecture based algorithm provides automatic and robust segmentation of the cardiac chambers and myocardium in CCTA images. The output of the model is an automatic multi-class segmentation of the LV myocardium, LV cavity, RV, LA, and RA across the entire cardiac cycle. The model reaches a performance of a median Dice coefficient of 0.908. Try out this algorithm with your own data!🚀 Upcoming challenges and running challenges¶
As summarized in our previous newsletter, there are two types of challenges on our platform. A type 1 challenge is where both the training data (including ground truth) and testing data (excluding ground truth) are made publicly available, with only the ground truth files for testing data to be kept secret. Participants are expected to upload predictions of the publicly available testing data. But in a type 2 challenge, participants submit an algorithm as a solution. This algorithm is then run on a secret test set (uploaded to an archive by the challenge admins). With the introduction of type 2 challenges, most challenges are moving to become type 2 challenges.
🥅 Goal: An AI challenge to raise awareness about the problems of distributional shift, robustness, and uncertainty estimation, and to identify new solutions to address them with two different challenge tracks:
- To develop robust MS lesion segmentation models in 3D MRI of the brain
- To develop uncertainty-aware and robust models to predict energy consumption of maritime transport to reduce the carbon footprint of global supply chains
✍️ Register: Accepting submissions from September 15th
⏰ Deadline: The development phase ends on February 28th, 2023
🏆 Prize:
- A villa in Greece for a week (shared between the teams) and an Antikythira Mechanism (each team)
- A villa in Greece for 4 days (shared between the teams) and an Antikythira Mechanism (each team)
- A replica Antikythira Mechanism (each team)
🏆 Leaderboard of finished challenges¶
The goal was to automatically segment tumor lesions in whole-body FDG-PET/CT on a large-scale database of 1014 studies of 900 patients (training database) acquired on a single site focusing on accurate and fast lesion segmentation and the avoidance of false positives (brain, bladder, etc.) The overall winners are Ziyan Huang with Jin Ye, Mingjian Li with Yige Peng, and Jia Zhang, with Yukun Huang and Yuhang Shi. Congratulations!
The goal was to segment the Intracranial Hemorrhage region in Non-Contrast head CT (NCCT). The segmentation performance is evaluated in four aspects following: Dice coefficient, Hausdorff distance, relative volume difference, and the normalized surface Dice. The winners are Qinghui Liu, Andriy Myronenko, and Dong Yang. Congratulations!